Amazon Sagemaker
Amazon SageMaker is a fully-managed platform that enables developers and data scientists to quickly and easily build, train, and deploy machine learning models at any scale. With Amazon SageMaker, all the barriers and complexity that typically slow down developers who want to use machine learning are removed. The service includes models that can be used together or independently to build, train, and deploy your machine learning models.
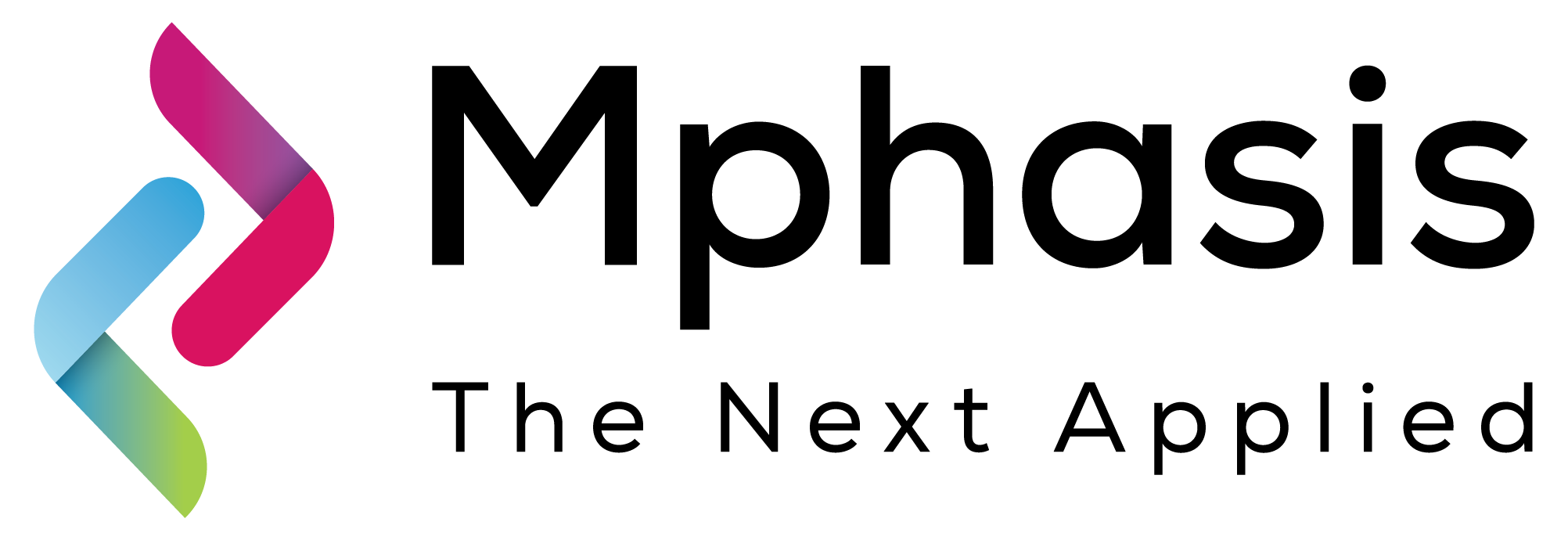
Task-tuned embeddings for search
By:
Latest Version:
v1
Solution enhances the search for specific business processes by accounting for language and intents of different user roles in the domain.
Product Overview
In search engines, the way search is conducted varies based on purpose, information needed, and the available resources within a business process. This solution uses state-of-the-art GenAI techniques and Anthropic Claude to customize the embedding LLM model to capture the nature of questions and domain semantics. Alignment of embeddings using finetuned LLMs improves the rankings of relevant content in search results. The inputs are raw documents (most formats of documents containing text and images) along with metadata such as: role of the user intendnig to use the search engine, user intentions, description of the data and usage of the data. For example, a maintenance engineer, searching thourhg maintenance logs and troubleshooting content. The embedding model is fine-tuned on this dataset and can be used at inference to vectorize the final desired corpus and incoming queries for search. Please note that the product requires an AWS bedrock anthropic Claude V2 model subscription.
Key Data
Version
By
Categories
Type
Algorithm
Highlights
The GenAI solution is intended to be used as part of a search and retrieval workflow. The embeddings generated from a fine-tuned LLM given the domain-specific raw documents can be useful to embed your documents to a vector database and vectorize incoming search queries. The solution can accept all format documents and the output is a zip file with embedding in excel format.
The input can be of any data format including docx, pdf, ppt, images, xlsx, etc., and requires no data preparation. only raw documents along with the user intent and data description to understand the data and the user requirement. The generated output captures the type of questions and also the contextual meaning of keywords. The users require AWS credentials for an account that has a Bedrock - Anthropic-Claude-v2 model subscription.
Mphasis DeepInsights is a cloud-based cognitive computing platform that offers data extraction & predictive analytics capabilities. Need customized Machine Learning and Deep Learning solutions? Get in touch!
Not quite sure what you’re looking for? AWS Marketplace can help you find the right solution for your use case. Contact us
Pricing Information
Use this tool to estimate the software and infrastructure costs based your configuration choices. Your usage and costs might be different from this estimate. They will be reflected on your monthly AWS billing reports.
Contact us to request contract pricing for this product.
Estimating your costs
Choose your region and launch option to see the pricing details. Then, modify the estimated price by choosing different instance types.
Version
Region
Software Pricing
Algorithm Training$4/hr
running on ml.p2.xlarge
Model Realtime Inference$0.50/inference
running on any instance
Model Batch Transform$4.00/hr
running on ml.p2.xlarge
Infrastructure PricingWith Amazon SageMaker, you pay only for what you use. Training and inference is billed by the second, with no minimum fees and no upfront commitments. Pricing within Amazon SageMaker is broken down by on-demand ML instances, ML storage, and fees for data processing in notebooks and inference instances.
Learn more about SageMaker pricing
With Amazon SageMaker, you pay only for what you use. Training and inference is billed by the second, with no minimum fees and no upfront commitments. Pricing within Amazon SageMaker is broken down by on-demand ML instances, ML storage, and fees for data processing in notebooks and inference instances.
Learn more about SageMaker pricing
SageMaker Algorithm Training$1.125/host/hr
running on ml.p2.xlarge
SageMaker Realtime Inferencenot available
running on ml.p2.xlarge
SageMaker Batch Transformnot available
running on ml.p2.xlarge
Algorithm Training
For algorithm training in Amazon SageMaker, the software is priced based on hourly pricing that can vary by instance type. Additional infrastructure cost, taxes or fees may apply.InstanceType | Algorithm/hr | |
---|---|---|
ml.p2.xlarge Vendor Recommended | $4.00 | |
ml.p2.8xlarge | $4.00 | |
ml.g4dn.xlarge | $4.00 | |
ml.g4dn.2xlarge | $4.00 |
Usage Information
Training
Usage Methodology for the algorithm:
1) The input must be 'Input.zip' file.
2) The zip file should contain Input file which includes a config.json file and train_doc folder.
3) The config.json file should contain the aws account credentials with bedrock model subscription.
4) The hyperparameters keys named as 'data_description', 'user_intention' and 'no_of_questions' must be define in config file.
5) The train_doc folder contain all the raw input documents (all format accepted).
6) check the instructions and sample endpoint in the sample jupyter file provided.
Channel specification
Fields marked with * are required
training
*Input modes: File
Content types: application/zip, application/gzip, text/csv, application/json
Compression types: None, Gzip
Model input and output details
Input
Summary
The inference pipeline requires:
- A Input.zip file (case sensitive) which include a .csv file named as 'test.csv'.
- The 'test.csv' contain only one column called 'text'.
Input MIME type
application/zip, application/json, application/gzip, text/csvSample input data
Output
Summary
The output will a zip file named 'output.zip' which contains an csv file named 'embeddings.csv' which includes the embeddings for the input text.
Output MIME type
application/zip, application/gzip, text/csv, application/jsonSample output data
Sample notebook
Additional Resources
End User License Agreement
By subscribing to this product you agree to terms and conditions outlined in the product End user License Agreement (EULA)
Support Information
Task-tuned embeddings for search
For any assistance reach out to us at: https://www2.mphasis.com/AWS-Marketplace-Support-LP.html
AWS Infrastructure
AWS Support is a one-on-one, fast-response support channel that is staffed 24x7x365 with experienced and technical support engineers. The service helps customers of all sizes and technical abilities to successfully utilize the products and features provided by Amazon Web Services.
Learn MoreRefund Policy
Currently, we do not support refunds, but you can cancel your subscription to the service at any time.
Customer Reviews
There are currently no reviews for this product.
View allWrite a review
Share your thoughts about this product.
Write a customer review